Enhance Machine Learning Models with Databricks Integration
Solution Overview
Together, Tecton and Databricks provide a simple and fast path to building and serving production-grade features to support a broad range of machine learning applications, including fraud detection, recommendation systems, dynamic pricing, search, and much more.
Available to all Databricks customers, Tecton acts as the central hub for ML features, allowing data teams to define features as code using PySpark, Python, or SQL and then automating production-grade ML data pipelines to generate accurate training datasets and serve freshly computed features online for real-time inference.
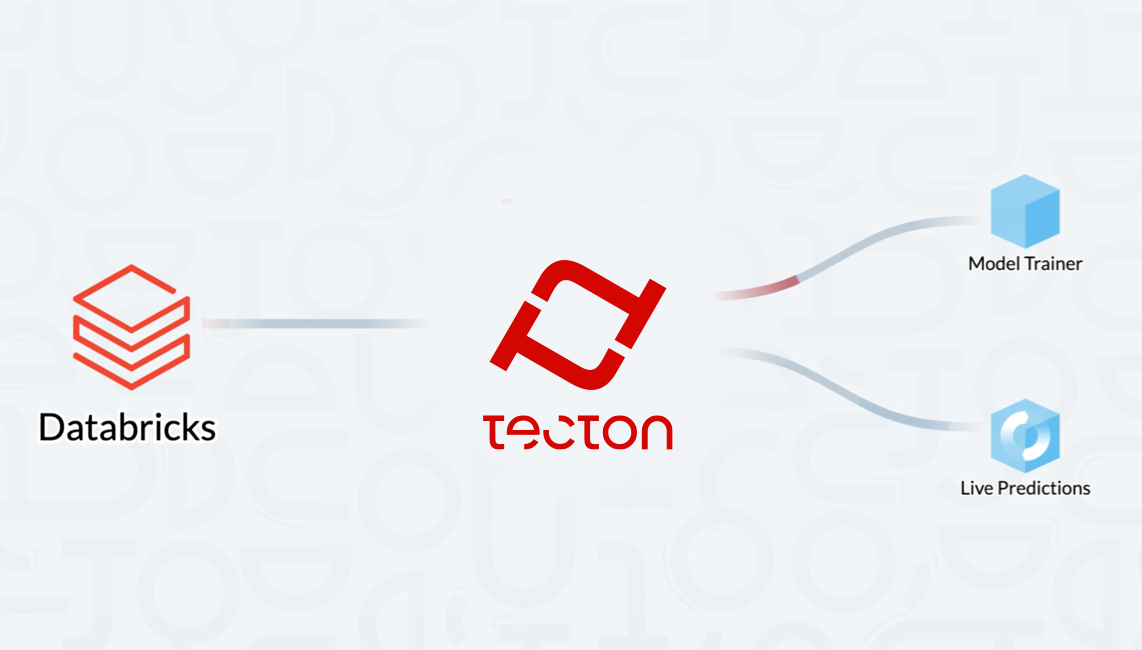
Key Benefits
Power
Build more powerful models by easily incorporating batch, streaming, and real-time data.
Speed
Deliver more business value from real-time ML applications in minutes rather than months.
Flexibility
Continuously improve and iterate on production ML models across teams and use cases.
As a net result of using the Tecton feature store, we’ve improved over 200,000 customer interactions every day. This is a monumental improvement for us.
Geoff Sims, Principal Data Scientist
Key Challenges of Production ML
Whether you’re building batch pipelines or already including real-time features in your ML initiatives, Tecton solves the many data and engineering hurdles that keep development time painfully high and, in many cases, predictive applications from ever reaching production at all, including:
- Training-serving skew
- Point-in-time correctness
- Productionizing notebooks
- Real-time transformations
- Melding batch + real-time data
- Latency constraints
- Data scientist and data engineering siloed workflows
- Limited discovery and re-use of features across teams
How it Works
Sitting on top of Databricks Delta Lake and leveraging the elastic scalability and power of the Databricks processing engine, Tecton’s feature platform enables data engineers and data scientists to build production-ready feature pipelines, and serve them at scale across teams, systems, and models, with only a few lines of code.
Under the hood, Tecton abstracts and automates the complex process that transforms raw data from batch or real-time sources into features used to train ML models and feed predictive applications in production. This process ensures data scientists can train models using historical features without worrying about point-in-time correctness or consistency with model serving. To run models in production, data engineers can rest assured that Tecton will serve only the latest features while maintaining high scale, high freshness, and low latency.
Managing the ML feature lifecycle with Tecton and Databricks not only ensures that feature materializations are always consistent, offline for training and online for inference, but that they are also stored in a searchable repository for easy sharing and re-use across teams and use cases.